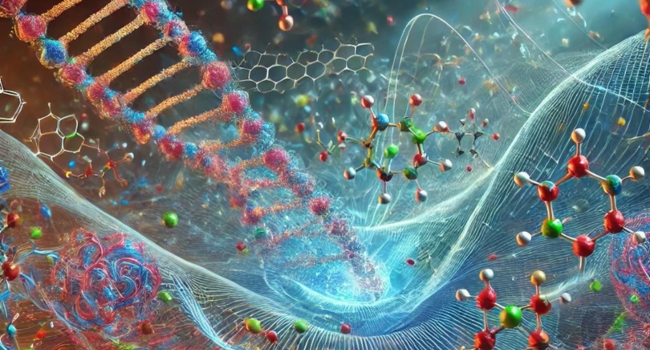
Predicting Small Molecule Interactions with RNA: A New Frontier in Drug Discovery
RNA, once considered merely a messenger between DNA and proteins, has emerged as a critical therapeutic target. Small molecules capable of binding to RNA can influence its structure and function, offering promising avenues for treating diseases such as cancer, viral infections, and genetic disorders. In this blog, we explore the tools and approaches used to predict RNA-small molecule interactions.
The Importance of RNA as a Target
RNA plays diverse roles in cellular processes, from coding proteins to regulating gene expression. Aberrant RNA structures or functions are implicated in various diseases, making them attractive targets for drug discovery. However, designing molecules that interact specifically with RNA poses unique challenges due to its structural complexity and dynamic nature.
Computational Tools for Prediction
Advances in computational biology have equipped researchers with tools to predict RNA-small molecule interactions efficiently. Key methodologies include:
Molecular Docking
Molecular docking simulates the interaction between small molecules and RNA, predicting binding sites and affinities. Tools like AutoDock and MOE are widely used for this purpose.
Machine Learning Models
Machine learning (ML) leverages large datasets of RNA structures and small molecule libraries to predict potential interactions. ML algorithms can identify patterns and properties that correlate with binding efficiency, accelerating the discovery process.
Molecular Dynamics Simulations
These simulations provide insights into the stability and dynamics of RNA-small molecule complexes over time, offering a detailed view of interaction mechanisms.
Databases and Resources
RCSB PDB: Provides 3D structural data of RNA and RNA-small molecule complexes. RNALigand: A curated database of RNA-binding small molecules. CHEMBL: Contains bioactivity data for small molecules interacting with RNA.
Challenges in RNA-Targeted Drug Discovery
Despite the progress, several challenges remain: High Flexibility: RNA’s dynamic nature complicates the prediction of stable binding sites. Off-Target Effects: Ensuring specificity to avoid interactions with unintended RNA molecules. Limited Structural Data: Fewer experimentally resolved RNA structures compared to proteins.
Future Directions
Emerging technologies are addressing these challenges: Cryo-Electron Microscopy: Providing high-resolution RNA structures for better predictions. AI-Driven Approaches: Deep learning models are increasingly accurate in predicting RNA-ligand interactions. Hybrid Methods: Combining computational and experimental techniques for validation.
Conlcusion
The ability to predict interactions between small molecules and RNA marks a significant step in the field of drug discovery. By overcoming existing challenges and leveraging innovative tools, researchers are poised to unlock RNA’s therapeutic potential. This area of study promises to revolutionize treatments for complex diseases, bringing hope to millions of patients worldwide.